CAPT Seminar - Ass. Pr. Georgios Fanourgakis Jeudi, 16h00 - Amphi IPREM
Le 1 juin 2023 à 16h00
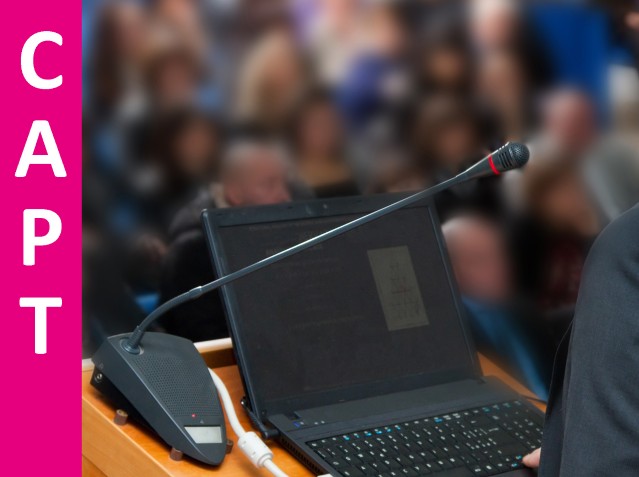
Ass. Pr. Georgios Fanourgakis
(Laboratory of Quantum and Computational Chemistry,
Aristotle University of Thessaloniki, Thessaloniki)
"Machine Learning Applications
in Studying Nanoporous Materials"
Abstract:
Due to their exceptional host-guest properties, nanoporous materials, such as Metal-Organic Frameworks (MOFs) are promising candidates for storage of various gases with environmental and technological interest. The discovery of new materials with desired properties may have an important impact in several scientific and technological fields. The number of these materials that can be potentially synthesized by combining various structural building blocks is enormous, while their experimental synthesis and characterization is a time-consuming and expensive process. So far, only few thousands of them have been experimentally synthesized and even less have been evaluated for their gas capacity. Molecular modeling and simulations are invaluable tools, extensively used over the last two decades for the study of various properties of MOFs. Despite the accuracy and efficiency of molecular simulations, the high-throughput screening of the enormous number of MOFs is also beyond present computer capabilities. Application of machine learning (ML) methods for the study of gas adsorption in nanoporous materials represents a promising and efficient alternative of the previous approaches. In principle, a ML predictive model can almost instantly provide predictions for millions of these materials. During the presentation I will discuss a number of challenges that should be addressed before ML methodologies replace or significantly reduce the need of employing the previous traditional approaches. These challenges are related to the description of the system in a manner understandable by the ML algorithms (a.k.a. descriptors or futures), the data needed for the proper and efficient training of the ML algorithms, as well as the inherent limitation of ML methods to provide reliable predictions for cases far from the already known ones. Our recent efforts to address and overcome these challenges [1-5] will be presented.
References
[1] G. S. Fanourgakis, K. Gkagkas, E Tylianakis, E. Klontzas, and G. Froudakis, “A Robust Machine Learning Algorithm for the Prediction of Methane Adsorption in Nanoporous Materials” J. Phys. Chem. A 123, 6080 (2019)
[2] G. S. Fanourgakis, K. Gkagkas, E Tylianakis and G. Froudakis, “A Universal Machine Learning Algorithm for Large Scale Screening of Materials”, J. Am. Chem. Soc. 142, 3814 (2020)
[3] G. S. Fanourgakis, K. Gkagkas, E Tylianakis and G. Froudakis, “A Generic Machine Learning Algorithm for the Prediction of Gas Adsorption in Nanoporous Materials” J. Phys. Chem. C, 124, 7117 (2020)
[4] G. S. Fanourgakis, K. Gkagkas, E. Tylianakis and G. E. Froudakis, “Fast Screening of Large Databases for Top Performing Nanomaterials using a Self-Consistent, Machine Learning based Approach” J. Phys. Chem. C, 124, 19639 (2020)
[5] G. S. Fanourgakis, K. Gkagkas and G. E. Froudakis, “Introducing artificial MOFs for improved
machine learning predictions: Identification of top-performing materials for methane storage” J. Chem. Phys. 156, 054103 (2022)